Tag Archives: Bag of words
BoW to BERT
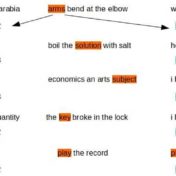
Word vectors have evolved over the years to know the difference between “record the play” vs “play the record”. They have evolved from a one-hot world where every word was orthogonal to every other word, to a place where word vectors morph to suit the context. Slapping a BoW on word vectors is the usual way to build a document vector for tasks such as classification. But BERT does not need a BoW as the vector shooting out of the top [CLS] token is already primed for the specific classification objective
Word Embeddings and Document Vectors: Part 2. Classification
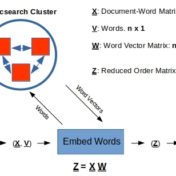
In the previous post Word Embeddings and Document Vectors: Part 1. Similarity we laid the groundwork for using bag-of-words based document vectors in conjunction with word embeddings (pre-trained or custom-trained) for computing document similarity, as a precursor to classification. It seemed that document+word vectors were better at picking up on similarities… Read more »
Word Embeddings and Document Vectors: Part 1. Similarity
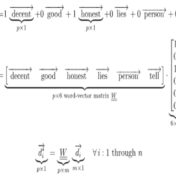
Classification hinges on the notion of similarity. This similarity can be as simple as a categorical feature value such as the color or shape of the objects we are classifying, or a more complex function of all categorical and/or continuous feature values that these objects possess. Documents can be classified… Read more »