Attention as Adaptive Tf-Idf for Deep Learning
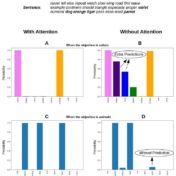
Attention is like tf-idf for deep learning. Both attention and tf-idf boost the importance of some words over others. But while tf-idf weight vectors are static for a set of documents, the attention weight vectors will adapt depending on the particular classification objective. Attention derives larger weights for those words that are influencing the classification objective, thus opening a window into the decision making process with in the deep learning blackbox…